Jio Platforms Launches Jio Brain, an AI and ML Platform for Enterprises
Leading telecom and tech company Jio Platforms recently unveiled Jio Brain. It is an artificial intelligence (AI) and machine learning (ML) platform for businesses. Jio Brain seeks to simplify the deployment of ML tools across telecom networks, enterprise environments, and industry-specific IT setups in everyday operations. It seeks to emphasize integrating 5G capabilities. Jio Brain’s enterprise-ready and mobile-ready large-language model (LLM) as a service feature. This enables its clients to take advantage of generative AI features, which is one of its main selling points. With over 500 data APIs and Representational State Transfer (REST) APIs, the cloud-native platform enables businesses to develop ML services tailored to their specific requirements.
Industry’s first 5G integrated ML platform
Aayush Bhatnagar, Senior Vice President of Jio, made the announcement. According to him, hundreds of engineers worked on the project for two years before Jio Brain was developed. The platform was described as “Industry’s first 5G integrated ML platform” in a document that was included with the announcement.
According to Bhatnagar, the platform was created following two years of research and development work by hundreds of engineers. He highlighted Jio Brain’s transformative potential. He said it will help develop new 5G services, transform businesses, and optimize networks. Furthermore, it will pave the way for the development of 6G, where machine learning will play a major role. With this unveiling, Jio is demonstrating its dedication to driving the enterprise application of AI and ML.
Read More: Nazara Tech’s Nodwin Gaming Acquires Comic Con India for Rs 55 Cr
Jio Brain
The platform is essentially an AI and ML-powered system. It provides enterprises with automation and possibly even generative AI features. It helps them run their operations more quickly and effectively. The service describes itself as “industry agnostic” and states that it provides many features. LLM as a Service, sophisticated AI features for text, images, videos, documents, and voice, a cloud-native solution with plug-and-play architecture, data integration capabilities, numerous AI/ML embedded mobile and web applications, and more are just a few of Jio Brain’s noteworthy products.
Jio Brain’s AI and ML services
Businesses can use Jio Brain to handle tasks related to natural language processing (NLP) and artificial intelligence (AI). These includes text-to-music, text-to-image and video, speech-to-speech, speech-to-text, and image-to-video generation. Jio Brain exhibits adaptability to managing a diverse range of applications. Its extensive feature set makes it an effective tool for businesses. It tries to improve productivity and creativity throughout its whole operation. The platform makes code generation, debugging, explanation, and optimization easier. Jio Brain also includes essential machine learning features like feature engineering, ML chaining, hyperparameter tuning, and more that can be used as a stand-alone service or in combination.
Crucially, Jio Platforms stated that it would be willing to work with researchers in AI and ML to make improvements. Jio’s dedication to collaborative innovation demonstrates its understanding of the dynamic nature of AI. Moreover, it also showcases the need to constantly push boundaries. Jio Brain seeks to remain at the forefront of technological developments in the AI and ML space by establishing partnerships with researchers. Although the announcement offers an overview of Jio Brain’s capabilities, the company has not provided a launch date for the platform. This gives the tech community cause for excitement and conjecture as businesses and industry insiders alike eagerly anticipate Jio Brain’s official launch and the revolutionary effects it may have on the market for business AI and ML solutions.
Read More: Publicis Groupe Plans €300 million AI Investment, Unveils CoreAI Platform
Reliance’s Recent Move Toward AI
Reliance recently revealed a collaboration to develop a sizable language model suited to India’s multifaceted linguistic environment. This initiative, in partnership with NVIDIA, aims to advance AI technologies for a range of applications, including drug discovery, chatbots, and climate research. In this project, NVIDIA will provide AI supercomputer technologies to Reliance Jio, the Reliance Group’s telecom subsidiary. These include networking solutions, graphics processing units (GPUs), CPUs, AI operating systems, and frameworks that are necessary for creating sophisticated AI models.
Reliance’s Latest Endeavors
Jio’s chair, Akash Ambani, recently revealed that the company is collaborating with IIT Bombay on the BharatGPT project. This comes also after it was revealed that Reliance and NVIDIA are collaborating to use GH200 GPUs in India to create AI models. Jio is developing its operating system (OS), especially for televisions in addition to these initiatives. By improving user interaction and engagement on Jio devices, this operating system seeks to bolster the company’s services throughout its ecosystem.
In conclusion, the introduction of Jio Brain by Jio Platform marks a major advancement in the application of ML and AI technologies in business settings. Jio Brain can completely change how businesses approach efficiency, innovation, and automation. This is because of its emphasis on 5G integration, customization, and cooperation with researchers. The excitement surrounding Jio Brain is growing as the tech community waits for more information and a confirmed launch date. This is a significant development in the changing landscape of AI and ML platforms for businesses.
Read More: Babita Baruah Joins VML India as CEO as Shams Jasani Steps Down
Google Cloud Introduces New Generative AI Tools for Retailers
On the eve of the annual convention of the National Retail Federation, Google unveiled several new generative AI tools intended to improve the online shopping experiences and other retail operations of retailers. The tools make use of artificial intelligence technologies that are generative. They are made to make the implementation of chatbots and AI easier, enhance search, and produce more customized shopping experiences. One of these new products is an AI-powered chatbot that merchants can incorporate into their mobile apps or websites.
The most recent illustration of generative AI’s expanding impact in the retail sector is found in Google Cloud’s products. Retailers can modernize operations, personalize online shopping, and change in-store technology rollouts with the aid of these generative AI-powered technologies. These virtual representatives can converse with clients and make recommendations for products based on their likes.
Google Cloud unveils new generative AI tools for retailers
In addition, tools for improving retailers’ customer support systems and streamlining their product cataloging procedures are included in Google’s recently released AI products. In addition to e-commerce, physical stores are receiving new artificial intelligence capabilities via Google Distributed Cloud Edge, an already available hardware and software package.
All these areas seem to have a great deal of room for improvement. After using virtual assistants, only about one-third of customers are happy with them, and almost 20% say they wouldn’t use them again. Customers are still very interested in using AI, though. Eighty percent of those who haven’t used the technology for shopping would like to give it a try. The majority, or five9%, would like to use AI applications while they shop.
Read More: Imagine Communications Join Forces with Google Ad Manager
New generative AI tools
Google’s offerings are aimed squarely at these issues. The new tools include:
Conversational Commerce
Similar to brand-specific ChatGPT, Conversational Commerce facilitates the joining of chatbots on websites and mobile apps. The salespeople converse with customers in plain language and make customized product recommendations depending on each person’s preferences. When it comes to products, they can have “helpful and nuanced” conversations with customers. Moreover, it offers recommendations based on their preferences.
Catalog and content Enrichment toolset
Google Cloud’s new Catalog and Content Enrichment toolset, which uses GenAI models—including the previously mentioned PaLM and Imagen—to automatically generate product descriptions, metadata, categorization suggestions, and more from as little as a single product photo, complements the Conversational Commerce Solution. Additionally, retailers can use the toolset to create new product images from pre-existing ones. Furthermore, it can also leverage product descriptions as the foundation for AI-generated product images.
For example, when a customer is looking for a formal dress for a wedding, a virtual agent can talk to them and offer customized product options based on their preferences for colors, the type of venue, the weather, complementary accessories, and price range. Importantly, rather than taking months, retailers can use these advanced conversation AI agents in a matter of weeks. This new solution can be integrated into a retailer’s current catalog management software or run on the Vertex AI platform on Google Cloud.
Read More: Google’s Third-Party Cookies Deprecation Rolls Out Today
Vertex AI
Additionally, Vertex AI Search for retail, a product from Google Cloud, has a new LLM capability. It provides retailers with natively embedded Google-quality search, browse, and recommendation capabilities on their unique product catalog and shopper search patterns. With the addition of new large language model (LLM) capabilities, Vertex AI Search enables sellers to tailor an LLM to their specific catalog and the search habits of their customers. By better ranking possible products as a fit for any given search term, it can give users more relevant search results.
Customer Service Modernization
Customer Service Modernization combines chatbots with an existing retailer’s CRM data. It enhances self-service, recommends products, sets up appointments, monitors order status, and more.
Google Distributed Cloud Edge
To lower IT expenses and resource commitments related to retail GenAI, Google unveiled the Google Distributed Cloud Edge. It is a managed self-contained hardware kit designed specifically for retailers. It is intended to facilitate retailers’ use of AI in places with spotty or nonexistent internet. Store analytics, frictionless checkout, and streamlined mission-critical store operations are currently its main use cases. The edge cluster, which powers customers’ GenAI apps, is said by Google to be compatible with a variety of retail spaces, including convenience stores, gas stations, fast-casual restaurants, and grocery stores. It comes in a range of sizes, from single-server to multi-server configurations.
Here’s what they said
Carrie Tharp, vice president of Strategic Industries, Google Cloud said,
In only a year, generative AI has morphed from a barely recognized concept to one of the fastest-moving capabilities in all of technology and a critical part of many retailers’ agendas. With the ability to accelerate growth, boost efficiency, fuel innovation, and reduce toil, generative AI solutions are ready to be deployed now, and Google Cloud’s recent innovations can help retailers recognize value in 2024.
Read More: Google Launches Google Ads Data Manager for First-Party Data
Yahoo Spins Out its Enterprise Engine Vespa for AI-Scaling Excellence
Yahoo announced the spin-out of Vespa, its enterprise AI-scaling engine to increase the reach of its technology platform. Vespa, which makes extensive use of data and AI online, will be a distinct and independent company. Since 2017, Vespa, a division of Yahoo, has served a range of external clients’ AI requirements, including Spotify, Wix, major financial institutions, and others. Searching through millions of documents inside a large enterprise, providing superior data-driven online services, or enabling scalable AI-based language apps are a few examples. 150 essential applications that are crucial to the business’s operations are supported by Vespa technology. These programs are necessary for real-time content personalization across all of Yahoo’s pages and for managing targeted advertising inside one of the world’s biggest advert exchanges.
What is Vespa?
In 1997, Vespa debuted as a cutting-edge search engine. Later, as a result of the Overture acquisition in 2003, it joined Yahoo. Vespa went open-source in 2017 and started providing services to outside customers in 2021. Through the spin-out, Yahoo will have a share in the new company and a director seat on its board.
Vespa – Yahoo’s spin-out
The spin-out intends to increase Vespa’s technology platform’s usability at a time when retrieval-augmented generation (RAG) requires the company’s artificial intelligence (AI) processing. With around 150 technology apps supporting close to one billion users, Yahoo will continue to be Vespa’s top customer and strategic investor. These programs process 800,000 inquiries every second, which is astounding. Vespa will also keep powering Yahoo’s internal solutions for search, dynamic recommendations, and providing users with targeted adverts and content.
Read More: Microsoft Advertising Enhances Search and Ads with Generative AI
Benefits of entity spin-out
Vespa will be able to benefit the rest of the globe greatly if a corporation is built around it. Additionally, it will enable businesses already reliant on Vespa to take advantage of cloud service efficiencies. More businesses will use it to solve online AI and big data concerns. Vespa will be able to quicken the creation of new features, enabling its users to produce even better solutions more quickly and inexpensively. Deploying on a cloud service or keeping with an open-source distribution are both possible options.
Thanks to its decade-long work on fusing AI and large data online, Vespa delivers features and scale that exceed any comparable solution. The demand for a platform that offers a solid basis for these solutions has never been greater as the world begins to use current AI to solve actual business problems online.
Here’s what they said
Jim Lanzone, CEO of Yahoo said,
Vespa has been a critical component to Yahoo’s AI and machine-learning capabilities across all of our properties for many years. While remaining Vespa’s biggest customer and a key investor, we’ll continue to leverage all that Vespa has to offer while simultaneously creating a new business opportunity that allows other companies to harness its technology as an independent entity.
Jon Bratseth, CEO of Vespa added,
Given Yahoo’s incubation and advancement of the technology over the years, now is the time to spin out Vespa and allow other companies to take advantage of Vespa Cloud in a meaningful way. With Yahoo’s continued investment and support, Vespa will be able to maintain its position as one of the largest and most sophisticated machine learning and database management platforms globally.
Read More: The AI Search War: Microsoft & Google Compete for Search Engine Leadership
Media.Monks Unveils AI Integration for Customized Content
S4 Capital’s solely digital operational brand, Media.Monks is introducing an AI product. It will integrate generative AI and machine learning into its software-powered production system. Additionally, it will support the delivery of highly customized content to customers in new media types. At the 2023 International Broadcasting Convention (IBC), NVIDIA, Adobe, and Amazon Web Services will present additional information on Media.Monks. The company uses strong software platforms, powerful GPUs, and cutting-edge networking technology to choose highlights from live transmission. Additionally, it will deliver customized information to certain online audiences. Brands and customers are expected to receive more personalized experiences, thanks to the partnership with NVIDIA.
AI Integation in Software-powered production system
MediaMonks’ software-driven production system is built to function remotely with a staff that is dispersed across. The method offers versatility by doing away with the requirement for broadcast appliances that are only used once. It also provides full-service consulting, production, and integration services. The solution captures live content on the spot and sends it to remote teams. Developed locally, through Amazon Web Services, it integrates edge ecosystem standards. It can easily adjust to the needs of delivering multi-format content across a range of new media formats, devices, and platforms. This method of live broadcasting reduces greenhouse gas emissions significantly.
- A geographically dispersed workforce and cloud redundancy further improve reliability. Additionally, it eliminates the requirement for single-use broadcast appliances while cutting expenses by an estimated 50% or more when compared to standard broadcast settings. The live broadcast production workflow that won the Excellence in Sustainability Award considerably cuts greenhouse gas emissions.
- Software-defined production, which is now available as a production, consulting, and integration service, lowers costs from conventional broadcast set-ups by an estimated 50% or more while increasing reliability through cloud redundancy and dispersed global staffing.
- The system can be effectively installed in the cloud on Amazon Web Services (AWS) in a conventional or edge ecosystem, or it can be deployed locally to let a quick team capture information locally for dissemination to remote teams.
Read More: LightBoxTV Extends Range with Total TV Solution for CTV Campaigns
Dedication to sustainability
Additionally, Media.Monks highlight its dedication to sustainability by pitching its remote service as a substitute for conventional broadcasting infrastructure. The concept however will need significant investment in transportation and physical infrastructure. At the 2023 NAB Show, the company received a trophy for excellence in sustainability awareness for reducing greenhouse gas emissions related to live production and broadcast operations.
Finally, Media.Monks’ incorporation of generative AI into their software-powered production system illustrates its commitment to providing clients with customized content while lowering costs and having a smaller environmental effect. By utilizing cutting-edge technologies, Media.Monks seek to remain on the cutting edge of the changing broadcast industry and offer a tailored and engaging experience for both advertisers and customers.
Here’s what they said
Lewis Smithingham, SVP of Innovation at Media.Monks, said
Our goal is to deliver a more personalised experience for consumers and brands as efficiently as possible. Fans are increasingly craving personalised content they can watch on non-linear channels, so we’re using the latest GPUs, networking technologies and software platforms from NVIDIA and AWS to build upon our next-generation broadcasting solution and deliver the content people most want to watch.
Bob Pette, Vice President and General Manager of Professional Visualisation at NVIDIA, added
The future of broadcast is AI-powered and software-defined. Our collaboration with Media.Monks will help deliver a more engaging and personalized experience for brands and consumers.
Read More: Criteo’s Commerce Max DSP Unites Retail Media with General Availability
Zoom Faces Backlash As Revised AI Policy Raise Privacy Issues
Zoom, the video conferencing company that emerged in 2020 during the Covid epidemic, is receiving harsh criticism for its revised terms and practices. Following two changes to its AI approach, the corporation has announced that it will develop AI-powered tools using client information without user approval. Zoom claims complete authority over data collected during Zoom calls under the amended AI policy terms. Furthermore, they can reuse and share this data in any way they wish while adhering to the law to develop their artificial intelligence/machine learning frameworks. By doing so, they have failed to give users the choice to opt out.
As part of our commitment to transparency and user control, we are providing clarity on our approach to two essential aspects of our services: Zoom’s AI features and customer content sharing for product improvement purposes. Our goal is to enable Zoom account owners and…
— Zoom (@Zoom) August 7, 2023
Updated TOS with AI policy
Zoom revised its service terms in July. These policy revisions follow a public uproar over ethical implications of developing AI models with consumer data. According to the TOS, Zoom may collect and use “service-generated data” on product usage, telemetry, and inspections to train AI models. Customers agree to allow Zoom to access, utilize, gather, create, change, distribute, process, manage, and keep service-related data for any reason, including ML or AI training and algorithm tuning, without attribution. The TOS statement, on the other hand, fails to explain or clarify what Zoom means by service-generated data.
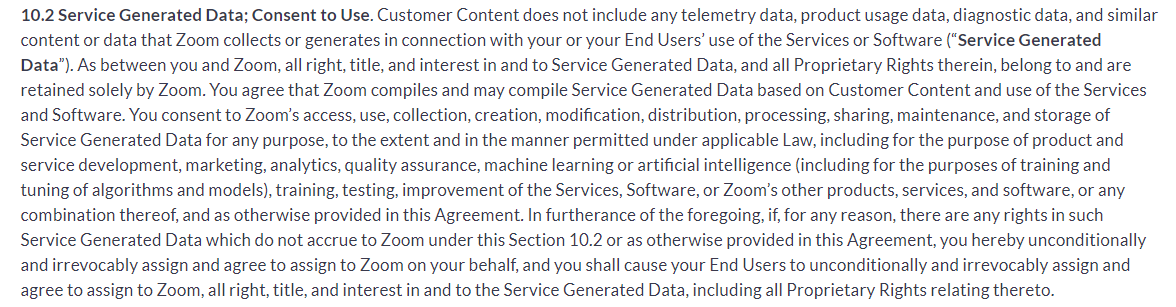
Image credit- Zoom
Read More: The AI Search War: Microsoft & Google Compete for Search Engine Leadership
Furthermore, according to revised policies, Zoom has obtained a long-term and global license that allows them to freely share, issue, access, utilize, stock, pass on, evaluate disclose, protect, extract, change, replicate, share, exhibit, distribute, translate, record, generate fresh versions, and manage customer content. A non-exclusive and royalty-free license allows sublicenses and transfers.
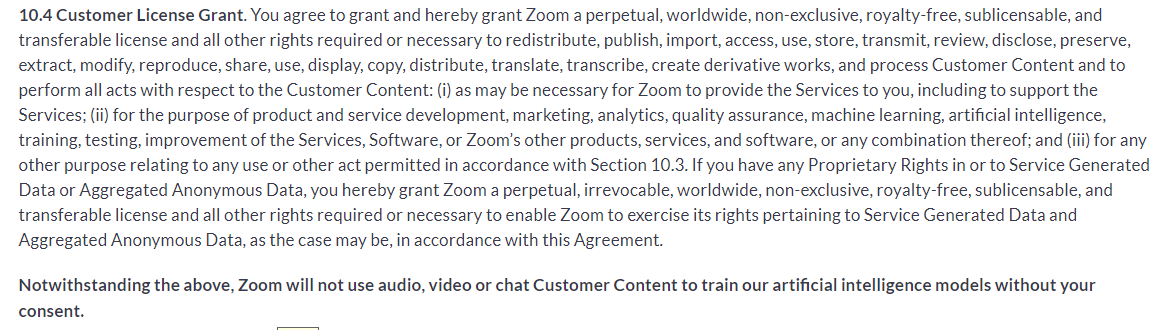
Image credit- Zoom
Zoom Responds
People express concerns about openness and privacy laws. Many people are concerned that Zoom’s updated restrictions may have significant ramifications in the telehealth and educational industries. These industries are governed by privacy laws. They also believe that it is critical to address AI model training issues using data gathered from recorded sessions.
Meanwhile, it denies using user information for AI training without first obtaining authorization. According to a blog post by Zoom, clause 10.2 is merely to enhance openness around data usage, to improve customer experience. This would entail studying usage trends such as peak hours in specific time zones to optimize the data center. This would improve video quality. Although Zoom has stated that it will not use audio, video, or chat content for training its models in the healthcare and educational sectors without client authorization, its terms of service appear inconsistent.
Smita Hashim, Chief Product Officer for Zoom writes,
An example of a machine learning service for which we need license and usage rights is our automated scanning of webinar invites/reminders to make sure that we aren’t unwittingly being used to spam or defraud participants. The customer owns the underlying webinar invite, and we are licensed to provide the service on top of that content. For AI, we do not use audio, video, or chat content for training our models without customer consent.
Read More: Battle of the Ads: Borzo Reveals Who Wins – Advertising Team or AI?
Zoom’s AI-powered assistant Zoom IQ contradicts TOS
A few months ago, Zoom introduced Zoom IQ, an AI-based assistant. This function, which summarizes conversation threads and produces automated answers to chat queries, is optional. However, Zoom IQ is automatically enabled. Users who do not change their settings give businesses permission to collect their data.
The other participants in the call receive a notice stating “Meeting Summary has been enabled” when a Zoom meeting starts with Zoom IQ enabled. Inputs and AI-generated content can be accessed from all users by the account holder. The company ties this to model training and product improvement. The participants have two options: accept the meeting or quit it. It is impossible to avoid sharing private information. In other words, someone else can give Zoom permission to use your data to create AI on your behalf.
A look at the future
Zoom, whose popularity surged during the Covid-19 outbreak, has received criticism for its privacy practices in the past. The business was hit with a $85 million lawsuit the year before due to a security flaw that let hackers access unidentified virtual meeting rooms. Making adjustments to its policies to remain relevant is unavoidable given the constantly changing AI landscape. In addition to OpenAI’s ChatGPT and Google’s BARD chatbot, other AI platforms have raised similar concerns. The results of Zoom’s recent changes to its terms and rules are uncertain. Will it further damage its well-established reputation or turn out well?
Read More: FTC Issues Notice to OpenAI over ChatGPT’s Privacy Data Breach
A Look Ahead: Convergence Of Linear TV And Digital TV Advertising
Digital and Linear TV advertising – two culturally and technologically distinct ecosystems – are merging to increase the effectiveness of marketing to consumers.
The shift from traditional linear television to connected television (CTV) and video-on-demand options has made it difficult for advertisers to reach mass audiences. Intriguingly, some of the same techniques used for converting television viewers to digital audiences can be applied to converting addressable digital inventory into non-addressable inventory.
Interesting Read: Connected TV Explained: The Essential Glossary Of CTV
Machine Learning and Data Automation
Advertising technology(adtech) companies are one of the first to adopt big data, machine learning, and artificial intelligence. One of the reasons is that websites and apps generate enormous data that advertisers can utilize to achieve their quantifiable goals. To leverage this, automation is the key aspect in performance advertising. It is the most feasible economically logical step for marketers. Thus, small and big ad tech companies have embraced machine learning on a large scale successfully to attract customers and increase ROI.
On the other hand, TV advertising continues using conventional methods and relies on billboard data to understand the advertising reach. Even though the data is modeled but it is not as responsive as the techniques used in digital advertising.
As machine learning is not compatible with small and medium-sized data sets, advertisers are using moving to other innovative methods for TV ads. Data-Driven Linear Purchasing(DDL) is gaining momentum as it combines the precision of digital and the reach of TV. It leverages automation and data to target audiences on national linear TV. The method may not be at par with machine learning but optimizing DDL campaigns require accurate prediction and efficient complex procedures.
Interesting Read: AdTech Vs MarTech: Let’s Settle This Once For All!
Performance Measurement
The performance measurement is similar between the two media. In contrast to digital advertising, where convergent events are used to train sophisticated and supervised learning models, TV advertising is more difficult to measure. Nevertheless, Savvy TV buyers have various other ways to improve the buy like web and app analytics, group surveys, and more.
Despite TV advertising embracing the data value, digital advertising has been heavily criticized for using excessive amounts of data. As a result, government and industry privacy regulations have eliminated many of the drivers of machine learning techniques including the individual-level identifiers used during transactional events and advertising exposures.
The Road Ahead For Advertising
What is in store for advertisers after the privacy regulations?
Advertising technology (AdTech) companies can develop technology to learn from data samples, analyze that data based on context, and apply those findings to power buyers and sellers.
There is still enormous digital data despite changes in privacy policies. Advertisers should concentrate on targeting based on context and so machine learning is likely to be part of any solution. However, in television advertising, data scientists will have to prudently balance the statistical outputs of the models. Identifying and targeting audiences will remain a challenge for the advertising industry. In the meantime, data scientists who meld the best technologies from digital and television advertising can deploy powerful and accurate targeting in a more privacy-conscious world.
Interesting Read: The Ultimate A-Z Glossary Of Digital Advertising!
Snapchat Advertising: New Multi-format Ad Delivery To Increase Reach
Snapchat globally rolls out an industry-first offering, ‘Multi-format delivery’, enabling advertisers to buy multiple ad formats in a single integrated ad set. It allows advertisers to optimize their campaigns toward their key objectives.
Multi-Format delivery supports all types of video ads, including Collection Ads, Commercials, and Snap Ads, and Story Ads. Snap plans on introducing self-serve augmented reality formats by the second quarter of 2022 for multi-format delivery. By doing so, it will help Snap introduce augmented reality advertising to more marketers.
Interesting Read: Mediabrands and Snap Inc Collaborates For A Mobile Video Measurement
Embrace The Machine Learning System
This multi-format delivery option is intended to help advertisers be more flexible with campaign setup, so Snap’s Auctions can handle the optimization-related work. As part of a set, Snap determines the best inventory to deliver across all formats.
As quoted by Adexchanger, Peter Naylor, Snap VP of sales for America said,
“When we allow machine learning to do its thing, we’re opening the aperture of possible inventory where an ad can run. It might be somewhere a brand hadn’t even conceived of before.”
Advertisers should not resist giving up manual control over the bidding and embrace the system to see the outcome for effective pricing.
Interesting Read: Snapchat Sees a 20% Plummet In Revenue Due To Apple’s Privacy Changes!
How Does It Work?
Within Snap Ads’ set up, a new ‘Multiple Formats Ad Set’ option will enable advertisers to bundle various creative options for placement across Snap’s suite of apps. It will enable advertisers to evaluate the efficiency of each format and assess its contribution to the success of their campaign.

Image Credit: Snap Inc

Image Credit: Snap Inc
A Snap ad set with multi-format delivery closely resembles a Facebook ad set: a collection of ads with similar settings for how, when, and where to show them. It will bring something new to the table, though when Snap will begin offering AR formats ad sets next year.
As reported by Adexchanger, Naylor said,
“This is going to unlock augmented reality for advertisers and show them that they can use it to accelerate their results in combination with everything else.”
He further added,
“Once we let machine learning reveal where the best inventory is against their goals, target or bids, they can focus more on the creative itself.”
Also Read: The Ultimate A-Z Glossary Of Digital Advertising!
A One-stop Guide On All You Ever Need To Know About AdTech In 2020
AdTech or Advertising Technology did around $800 Billion Worth Of Business In the US alone in 2019, making it one of the fastest-growing industries in the world.
Are you trying to understand ad tech? Just as advertising is the business of making advertisements, ad tech is the business of using technology to make advertisements faster, quicker, and efficient. The business is driven by powerful algorithms and data points. While it is not rocket science, but for the uninitiated, it can be challenging to understand what is ad tech and how its product and services work.
The ad tech industry fuels the global economy with big investments, employment, and ad spend. Digital advertising has reached new heights of complexity, with the rise of programmatic advertising, AI, and automated interactions between computer systems reducing human intervention. Today’s omnichannel ad campaigns reaching to different platforms all at once from publishers’ websites, mobile apps, social media to search engines. Campaigns using tailor-made and highly targeted ads to reach audiences. This process involves many participants- advertisers, publishers to third-party vendors. The technology used in advertising to store, manage, and deploy data is far more sophisticated.
This guide will give you a sneak-peek into the world of technological advertising and understand the growing ad tech industry. As you read further, you will understand the ever-changing ad tech ecosystem.
What is Ad Tech?
Ad Tech also is known as Advertising Technology covers a range of tools and software that can be helpful for brands and agencies to plan, strategize, and manage all digital advertising activities.
The AdTech ecosystem consists of two major entities – the advertiser (Demand-side) and the publisher(Supply-side).
On one hand, advertisers want to run effective campaigns and optimize their budgets to reach the target audience, gain customer insights, and measure ROI.
Whereas, on the other hand, publishers cater to the need of advertisers and generate revenue through ads by displaying ads on their publications like websites, apps, etc, increase ad impressions, bids for ad slots and visitor insights. These are significant factors that publishers need to consider to maintain the platform User Interface (UI).
Adtech helps advertisers and publishers achieve their goals in harmony by providing solutions that meet the demands of both parties. A few examples of AdTech platforms include Pubmatic, Adroll, MediaMath, SmartyAds, and many more.

Image Credit: MarTech Advisor
Programmatic Advertising Explained
After a brief understanding of ad tech, let’s step into the world of programmatic. You will come across concepts like programmatic advertising, Real-time bidding, and programmatic direct. Let’s discuss it:

Image Credit: Martech Advisor
- Programmatic Advertising Definition:
It is projected to be the game-changer for digital advertising. Programmatic automates the process of buying and selling online advertising space with the help of technology and data. This means, with the introduction of programmatic publishers, advertisers or agencies don’t have to sit across to discuss ad size, rates, et. Ad buying is done through algorithms and data insights.
- Programmatic Direct:
This a type of Programmatic digital advertising, where a publisher bypasses auction and reserves a portion or entire ad inventory for a particular buyer or advertiser at a fixed cost per mile. (CPM). Put simple, here the buyer and seller are known to each other and the ad placement is done programmatically.
- Real-Time Bidding (RTB):
Another type of programmatic digital advertising and also known as an open auction. RTB is when inventory prices are decided through an auction in real-time and open to both advertisers and publishers. This is the most feasible and preferable method of programmatic ad-buying because of scalability and flexibility.
The AdTech EcoSystem

Image Credit: Martech Advisor
The process of digital media buying is similar to the traditional media value chain except AdTech has multiple components in the ecosystem to keep the management of advertising campaigns easy for demand and supply-side platforms. Here are the key components of the AdTech supply chain:
1.Media agency: Responsible to allocate the advertiser’s expenditure budget across the channel. It is not involved in the creative aspect of ad campaigns.
2.Agency Trading Desk (ATD): Plans, buys, and manages ads across different platforms and is a set of services provided by the media agency.
3.Demand-side Platform (DSP): An essential platform for advertisers to buy, search, display video mobile ads. It enables advertisers to buy ad placements in real-time on the publisher websites made available by ad exchange and networks. Some of the DSP players are Simplifi, Smarty Ads, App Nexus, Double Click, and more.
4.Data Management Platform (DMP): DMP’s collect data from sources like websites, apps, social networks, campaigns, CRM’s, and more. Using AI and big data analytics to gather first and third-party data, advertisers, and marketers rely on them. DMP players are Lotame, Oracle Blue Kai, SAS data management and more
5.Ad Networks: The unsold inventory will be bought by ad networks from publishers and try to sell to advertisers using their technology. The popular programmatic advertising platforms for the ad networks are Taboola, Google Double Click Ad Exchange, Rocket Fuel, and more.
6.Ad Exchange: A dynamic platform to buy and sell ad impressions between advertisers and publishers without any intermediaries. Open X, App Nexus, Rubicon Project Exchange are examples of programmatic advertising platforms.
7.Supply Side Platform (SSP): The platform allows publishers to sell display, mobile ad impressions to potential buyers in real-time. Some of the key SSP players are MoPub, AerServ, App Nexus Publisher SSP, and more.
8.Ad Server: This platform is used by advertisers, publishers, ad networks, and ad agencies to run their campaigns. It determines which ad will be displayed on a website and also collect ad performances data such as clicks and impressions Double click for publishers, OPen X Ad server, Ad butler, and more are the examples.
Learn more: Programmatic Advertising Platforms in 2020: A Complete Guide
Is Programmatic advertising worth it?
The programmatic advertising statistics say it all. According to Zenith’s Programmatic Marketing Forecasts 2019, 69% of digital media will be programmatic in 2020.
- The total amount spent programmatically will exceed US$100bn for the first time in 2019, reaching US$106bn by the end of the year, and will rise to US$127bn in 2020 and US$147bn in 2021.
- 72% of digital media will be programmatic in 2021
- Ad spends growth is slowing down to 22% in 2019 due to industry challenges of privacy and supply-chain.
- Brands need to develop new targeting techniques using first-party data and customer data platforms in response to the ongoing death of the cookie.
Programmatic Display Advertising fastest-growing segment.
- The ascent of programmatic display advertising has been rapid. In 2012, only 10.4 % of global digital display spend was programmatic. However, it ballooned to 65.3% in 2019 and it is estimated that the share of programmatic display advertising will grow 69.2 5 and 72% in 2020 and 2021 respectively.
- How does it translate in dollars? In 2012, total digital ad spend was $37.8 billion and the programmatic display market was $3.9 million. Fast forward to today, digital display ad spend is $162.3 billion, out of which $106 billion is invested in programmatic display advertising. In 2021, global digital display ad spend is estimated to reach $204 billion, with $147.1 billion going to be programmatic share.

Image Credit: Marketing Charts
Programmatic marketing by country
One of the benefits of programmatic technology is it shows real-time data that helps companies take swift actions to adjust their strategy as per customer requirements. Digital marketers are considering buying programmatic media in-house due to its transparency. Programmatic has undergone massive growth in the following 6 countries out of which the UK and the US are the most advanced programmatic markets in the share of digital media.

Image Credit: Marketing Profs
As per eMarketer forecast, Programmatic ad spending will reach $59.45 billion in 2019, accounting for 84.9% of the US digital display ad market. It is estimated that 87.5%, or $81 billion, of all US digital display advertisements, will be bought via automated channels in 2021.

Image Credit: eMarketer
The above programmatic advertising statistics prove that the investment has increased Y-o-Y and marketers prefer programmatic advertising to buy digital display ads. Marketers are increasingly allocating their advertising budgets to digital advertising channels as it provides precise data that helps to reach customers effectively.
Learn more: 5 Programmatic Advertising Case Studies That Yielded Exponential Results
Artificial Intelligence can be leveraged in AdTech Industry
Artificial Intelligence(AI) and Machine Learning (ML) are two buzzwords in recent times. And why not, as it brings efficiency in whatever we do.
However, AdTech is a messy market now. Ironically, the good and bad part of AdTech is the abundance of data. True, we certainly have all the information to better understand the customers but most marketers aren’t aware of how to leverage the data and use it forward.
The way you advertise-is going to change extremely right before your eyes- thanks to Artificial Intelligence. Not all in the AdTech world have the analytical skills to evaluate the big data as not many are trained to use it and are misinterpreting them.
Adtech partnering with AI can help lower CPC prices, higher click-through rates (CTR), conversions, and better ROI. Let’s check out how AI can help the Adtech industry find better solutions in the following areas.
- AI in Ads Positioning
Developers don’t need to sit and determine which ad position will drive maximum revenue for the website. With the help of AI, employing machine learning algorithms study historical data to find relevant ads for the targeted user group.
Adtech has not used heatmaps previously but AI algorithms use them to learn where the visitors on the website are going and present them with the relevant ads. AI will help marketers to find the best ad positions by studying the maps in detail.
- AI in Ad Network Selection
There are many Ad Networks that provide different kinds of ads to websites owners and required to sort ads according to the websites. This is called Ad mediation and apt to earn high revenue for the websites.
By employing AI for ad optimization it reduces human effort by using a data-oriented approach that includes data, facts, and intelligence to make sure only relevant ads reach the end-user. Data will be user or website’s past history and facts will be website content, geo, and timing. Machine learning algorithms are employed to enhance the best ad-user match.
- Analytics
In the Adtech world, data analytics is not ‘taken seriously’ and publishers.are not happy about it. The AI-based approach will drive reporting and analytics to new levels.
Analytics will help publishers understand the content that drives the audience, placement of the CTA button to turn one time users into loyal users, and increase traffic. It will be a win-win situation for AdTech and parties- publishers, platforms, and users.
AI is the Future of Advertising
Today, digital advertising cannot exist without AI. Behind most online ads are the sophisticated delivery systems in place powered by AI. These systems place the ads before users, the coordination process happens in real-time and generally is automatic. It’s called programmatic advertising.’
According to eMarketer, 86.2% of all digital display ads will be bought via automated channels and nearly $19 billion in additional spending will enter programmatic display platforms between 2018-2020.
Also, 90% of mobile display ads are bought programmatically. On the other hand, AI also powers advertising products offered by Facebook and Google. In 2017, 90% of the new advertising business was captured by these firms.
In recent times, brands are under more pressure to deliver relevant, personalized, and contextual ads to individual customer preferences.
How AI makes Programmatic Advertising better
More companies are turning to AI for creating advertising relevance at scale.
For instance, if you want to advertise on Facebook,-an AI-powered algorithm determines the relevance of the score of your ad. This means that the score impacts the ad delivery directly and influenced by the experience of the ad delivery to Facebook users. Expect a low score if the ad is not liked or is irrelevant.
This decision is made by machine and is beyond your brand’s control independent of strategic or creative decisions
A marketing company like Phrasee launched an AI tool that writes Facebook and Instagram ads. The AI tool assesses a brand’s voice and copy, then the machine writes the ad that performs better than human-written ads. Recently, it helped reduce one client’s cost per lead by 31%. Another AI-powered tool is Albert that helps automate media buying, testing, and optimization. It enhances ad performance and delivers relevant ads to the right person. This shows that relevance at scale is possible in advertising.
Emerging Programmatic AdTech Trends 2020

Image Credit: MarketingToolbox
1. AI in Programmatic Advertising:
Technologies such as artificial intelligence(AI) and machine learning (ML)have involved programmatic ad buying or bid optimization. Programmatic campaigns are used by companies for more targeted net across platforms. By 2020, it is expected that there will be a shift towards automating technologies like AI and ML to get the most from data.
2. First Part Data Move Made Important by GDPR:
After the announcement of the General Data Protection Regulation (GDPR) in Europe, last year on cookie crumble or removal of third party cookies is gradually turning out to be beneficial. The regulations protecting the privacy of user data initially looked limiting to ad tech experts but is resulting in cleaner and more reliable data over time.
Learn more: Digital Advertising Industry Plans To Replace Cookies With First-Party Data
3. Digital Out Of Home (DOOH) and Mobile Location:
Digital DOOH combined with mobile location data has the potential to help marketers to drive conversions in the offline world. Integrated ‘home-to-out-of-home’ programmatic advertising approach provides a smooth experience to the customers.
4. Voice-activated Ads:
The adoption of voice-based to in-home smart devices has grown rapidly. Gartner predicted by 2020, 30% of web browsing sessions will be done through voice-first browsing. Amazon sold over 100 million Alexa-enabled devices in 2018 compared to 2017. A recent survey by VoiceBot.AI revealed 25% of respondents orders everyday household items through voice assistants followed by apparel and games and entertainment.
Programmatic advertising helps marketers to optimize these ad spaces across in-home smart devices, to on-app audio ad opportunities, and connect to consumers through in-store ads, ads in elevators and taxis, and more.
5. Wearables will enhance programmatic advertising:
Wearables collect data on location, lifestyle, health metrics, and more. The market penetration of smartwatches has grown multifold over the years and programmatic advertising is already making its way into this medium. For instance, it helps advertisers run banner promos to customers on their Samsung or Sony smartwatches. The wearable ecosystem has a huge potential to grow and programmatic adtech can bring greater opportunities.
6. 5G in programmatic advertising:
The high speed and no buffering will encourage the rise of more users to spend time on videos on mobile devices. It will enhance other technologies such as AR-enabled ad displays, VR without headsets, and innovative new digital outdoor mediums.
This will give programmatic advertising new opportunities to run more interactive ads without any lags across mediums. By 2024, the use of 5G in AdTech is predicted to grow to 1.4 billion.
7. Evolution of Personalization:
With Gen Z and Millennials- the biggest demographics -personalization is a priority as they like all things customized. Personalization in advertising is going to be inevitable as the choices of the new generation are different. Therefore, programmatic customization by advertisers is increasing offering personalized, relevant messaging to their target group.
8. Blockchains and Ads.xt:
Ad frauds are increasing over the past few years. A cybersecurity firm Cheq reports that ad fraud damages will touch $26 billion in 2020, $29 billion by 2021, and $32 billion the year after that.
The only way to handle the frauds is by bringing transparency in programmatic advertising. Blockchain and Ads.txt (an Interactive Advertising Bureau initiative – Authorized Digital Sellers) can help to remove unrequired middleman, domain spoofing, and verification of publishers and allow transactions using cryptocurrencies.
Learn More: Advertisers Look For Greater Transparency In Programmatic Ad Buying
9. Programmatic TV, podcasts and audio Ads set to grow:
The content on TV has changed drastically. There is a paradigm shift in TV viewing from cable TV to over-the-top(OTT) like Amazon Prime or Netflix via an internet connection.
Programmatic advertising has a larger role to play to ensure marketers get the best of both worlds. Programmatic TV is also going to get more important with its data-driven approach for buying and delivering ads.
Programmatic in podcasts and audio advertising is also growing. Apps like Spotify and Soundcloud are seeing more user acceptance and a new advertising landscape is being created for companies to monetize on.
10. Omnichannel Programmatic:
Forrester defines omnichannel marketing as ‘the practice of digitally sequencing advertising across channels, which is connected, relevant, and consistent with the customer’s stage in their life cycle.’ This is how programmatic advertising is going to be in 2020 and beyond.
A single marketing resource or an ad can be customized programmatically suiting various platforms through programmatic AdTech.
11. Agencies to work on outcome-based pay:
Discussions are making rounds to switch to an outcome-based remuneration model. With increasing ad frauds and agencies promise programmatic tech, advertisers fear how their budgets were used and where their ads placed. There was a lot of wastage in the space. Media buying companies started giving outcome-based remuneration more prominence. Gradually, advertisers would like to see the full cost chain of their programmatic buys, pushing agencies to outcome-based pay.
12. In House programmatic advertising v/s agency.
An IAB report suggests that nearly 40% executing programmatic trading via in house and 50% publishers also have an in-house model. This means advertisers are looking for more transparency, control of their ad strategies, and outcome.
It makes more sense to have an in-house team for strategizing programmatic ads and an agency partner for implementing parts of it instead of having a full-stack programmatic AdTech in house.
Wrapping up
Yes, Adtech is complicated but the best part is that it allows integrating the whole toolset into a single system. According to Zenith Media, the ad spends on digital media will reach $329 billion in 2021. However, there are major concerns and challenges -Ad Fraud, transparency, and privacy issues need immediate action.
There have been big changes and improvements over what advertisers and publishers used to have earlier but it still needs more work and their expertise to handle the challenges and resolve for good.
Amazon Tests Customer Service Chat bots With Original Dialogue Capability
Amazon takes one more step towards its mission of being ‘Earth’s most customer-centric company’ by working on commercial service customer chatbot to generate original dialogue in real-time using recent major developments in AI language.
The retail giant said that most text-based online customer service system includes automated agents that can handle simple requests. Generally, these agents are governed by rules – flow charts that have specific answers to particular customer input. If any other request that does not follow under the flowchart, the automated agent transfers the request to the human customer service representative. So, now the company is rolling out a separate consumer-facing chatbot that uses neural networks than rules to match human-authored response template to customer requests.
This project marks one of the first tests in the customer service market with modern and new natural language processing technology that researchers believe has the power to boost the progress in this field. This model has leading-edge systems like OpenAI’s GPT-2 that draws on massive training datasets and predictive texts to generate realistic dialogues.
Most chatbots in the market today despite technological advancements in machine learning still run on automation than AI. While Microsoft’s DialoGPT has used a new language for a generative chatbot before, they are yet to see a commercial application.
Jared Kramer, an applied-science manager on Amazon’s Customer Service Tech team wrote in a blog post,
“It is difficult to determine what types of conversational models other customer service systems are running, but we are unaware of any announced deployments of end-to-end, neural-network-based dialogue models like ours”
According to the research paper, Amazon is hoping to safeguard its generative model for a chatbot with Response-ranking AI. In this scenario, a generative AI will list down possible responses to customer requests and a neural network will select the most pertinent response. Each model is trained on approximately 5 million conversation-response pairs from around 350,000 past interactions related to specific customer service issues.
Presently, random trails consist of two types of customer service issues: return refund status and order cancellations. An internal metric is used to measure the success of the new AI chatbots. According to the metric, the new AI chatbots have significantly outperformed the old ones that account for the successful completion of the transaction and whether customers have to follow up within 24 hours.